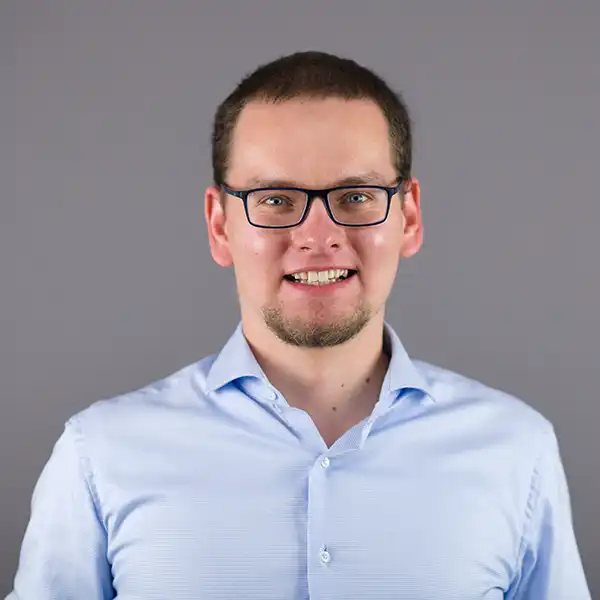
Tiny Tapeout is an educational project that makes it easy to get digital designs manufactured on a real chip. Project 12 is a state machine that generates signals for vehicle and pedestrian traffic lights at an intersection of a main street and a side street. A blinking yellow light for the side street is generated in the reset state.
Synchronization of sensor devices is crucial for concurrent data acquisition. Numerous protocols have emerged for this task, and for some multi-sensor setups to operate synchronized, a conversion between deployed protocols is needed. This paper presents a bare-metal implementation of a Tri- Level Sync signal generator on a microcontroller unit (MCU) synchronized to a master clock via the IEEE 1588 Precision Time Protocol (PTP). Cameras can be synchronized by locking their frame generators to the Tri-Level Sync signal. As this synchronization depends on a stable analog signal, a careful design of the signal generation based on a PTP-managed clock is required. The limited tolerance of a camera to clock frequency adjustments for continuous operations imposes rate-limits on the PTP-controller. Simulations using a software model demonstrate the resulting controller instabilities from rate-limiting. This problem is addressed by introducing a linear prediction mode to the controller, which estimates the realizable offset change during rate-limited frequency alignment. By adjusting the frequency in a timely manner, a large overshoot of the controller can be avoided. Additionally, a cascading controller design that decouples the PTP from the clock update rate proved to be advantageous to increase the camera’s tolerable frequency change. This paper demonstrates that a MCU is a viable platform to perform PTP-synchronized Tri-Level Sync generation. Our open source implementation is available for use by the research community.
Single pair Ethernet in combination with Ethernet endpoints provides a scalable basis for the direct control of sensors and actuators in zonal vehicle networks. As recently shown, this approach is also ideal for driving high-resolution light functions. The ability to transmit different parallel data streams to actuators opens a wide field for new applications. Here, we show a method for stabilising high-resolution light projections in driving operation. The stabilization of the light image is based on an inertial measurement unit that records vehicle movements in real-time. An algorithm in a central control unit continuously calculates correction values for the position and distortion compensation of the light distribution and sends this data to the lamp via Ethernet, preferably 10BASE-T1S. Two methods are combined in a proof of concept: predictive correction with video data rate and image shifting in the headlamp’s frame buffer at high frequency.
This paper presents a bare-metal implementation of the IEEE 1588 Precision Time Protocol (PTP) for network-connected microcontroller edge devices, enabling sub-microsecond time synchronization in automotive networks and multimedia applications. The implementation leverages the hardware timestamping capabilities of the microcontroller (MCU) to implement a two-stage Phase-locked loop (PLL) for offset and drift correction of the hardware clock. Using the MCU platform as a PTP master enables the distribution of a sub-microsecond accurate Global Positioning System (GPS) timing signal over a network. The performance of the system is evaluated using master-slave configurations where the platform is synchronized with a GPS, an embedded platform, and a microcontroller master. Results show that MCU platforms can be synchronized to an external GPS reference over a network with a standard deviation of 40.7 nanoseconds, enabling precise time synchronization for bare-metal microcontroller systems in various applications.
Real-time masking of vehicles in a dynamic road environment is a demanding task for adaptive driving beam systems of modern headlights. Next-generation high-density matrix headlights enable precise, high-resolution projections, while advanced driver assistance systems enable detection and tracking of objects with high update rates and low-latency estimation of the pose of the ego-vehicle. Accurate motion tracking and precise coverage of the masked vehicles are necessary to avoid glare while maintaining a high light throughput for good visibility. Safety margins are added around the mask to mitigate glare and flicker caused by the update rate and latency of the system. We provide a model to estimate the effects of spatial and temporal sampling on the safety margins for high- and low-density headlight resolutions and different update rates. The vertical motion of the ego-vehicle is simulated based on a dynamic model of a vehicle suspension system to model the impact of the motion-to-photon latency on the mask. Using our model, we evaluate the light throughput of an actual matrix headlight for the relevant corner cases of dynamic masking scenarios depending on pixel density, update rate, and system latency. We apply the masks provided by our model to a high beam light distribution to calculate the loss of luminous flux and compare the results to a light throughput approximation technique from the literature.
The reliable detection of vulnerable road users and the assessment of the actual vulnerability is an important task for the collision warning algorithms of driver assistance systems. Current systems make assumptions about the road geometry which can lead to misclassification. We propose a deep learning-based approach to reliably detect pedestrians and classify their vulnerability based on the traffic area they are walking in. Since there are no pre-labeled datasets available for this task, we developed a method to train a network first on custom synthetic data and then use the network to augment a customer-provided training dataset for a neural network working on real world images. The evaluation shows that our network is able to accurately classify the vulnerability of pedestrians in complex real world scenarios without making assumptions on road geometry.
Low-latency realtime video signal processing algorithms on embedded hardware platforms for high resolution LED-Matrix headlight systems. Our adaptive headlights prevent glare for drivers and pedestrians while increasing visibility and safety for nighttime driving.
Development of an Application Specific Instructionset Processor (ASIP) for computer vision applications like SIFT Feature extraction. We chose a wide horizontal datalevel parallelisation strategy with 1024 bits to enable pipelined processing of HD-images at high framerates.
Design Space exploration for FPGA-based hardware accelerators for object detection algorithms based on neural networks. Embedded Linux drivers for efficient DMA datatransfers over AXI4 on an FPGA-based SoC.
Institute of Microelectronic Systems: ims.uni-hannover.de
Google Scholar: scholar.google.com
ORCiD: orcid.org
Xing: xing.com
GitHub: github.com